背景
当前有两类实时任务需要频繁重启:第一类任务是,实时报表新增维度,此类任务中,Flink 消费 Kafka 实时数据,处理后按照维度聚合,使用聚合函数计算出指标后写入 StarRocks ;第二类任务是实时 ETL 任务,此类任务中,Flink 消费 Kafka 实时数据,经过字段提取、数据过滤再将结果回写 Kafka。这两类实时任务加字段(维度)需求较频繁、需求重复度高、需要重启程序,影响数据的准确性、及时性以及迭代速度较慢。因此,我们实现了基于配置化的方式,在不重启作业的情况下,快速满足新增字段(维度)的需求。
痛点
- 字段变更需要重启实时程序。由于字段变更的步骤较多,作业重启过程要持续 2 个小时,实时任务重启导致重复消费和部分任务需求方希望使用系统时间进行聚合,可能会出现数据重复计算以及时间错位等问题,重启期间数据准确性和及时性无法保证。
- 加字段(维度)需求多。场景一:算法需要实时地根据数据判断不同策略的转化好坏,从而选择最优策略进行广告投放。当算法新增策略时,就需要新增维度统计实时指标,该需求较为频繁;场景二:ADX 埋点和小说阅读器的其他埋点一起上报到 DRS 后,需要进行字段提取和特定埋点的过滤,因此在埋点结构发生变更时,就需要变更实时 ETL 任务提取新的字段,该场景更新频率较高。
- 迭代速度较慢。新增维度/字段涉及到以下流程:业务方通过 tapd 提需求 ---> 开发确认字段加工逻辑 ---> 代码实现 ---> 测试验证逻辑无误 ---> 停止任务,重建表同步历史数据,启动实时任务。整个流程走下来,大概需要一到两周。
技术方案
经过对上述应用场景痛点的介绍,相信读者会感觉到每新增一个维度/字段就重启一次程序的方式处理方式机械、迭代周期长、重启风险高。对此,行业内常用的解决方案有两种:
- 一种方式是使用 Flink CDC 实时接入规则流,和数据流进行双流关联后,按关联到的规则进行数据处理。这种方式的优点是规则能被实时处理,缺点是需要将状态设置为永不过期,并且重启时需要加载全量规则;
- 另外一种方式是采用维表存储规则,在接入实时数据时,使用自定义函数每隔一段时间拉取一次最新规则。这种方式的优点是实现相对简单,缺点是用户要综合考虑拉取频率和规则更新的及时性。考虑到当前应用场景中,规则可以先于数据添加,不要求高实时性,所以我们采用函数加载的方式定时拉取最新规则。下面我们将介绍如何使用配置化的方式,来避免此类机械、冗长高风险的实时程序重启流程。在介绍实现方案之前,我们先介绍一些基础知识,包括规则类型、规则是如何被存储的以及规则是如何被加载的。
规则配置使用
目前可以通过修改数据库添加和修改规则,后期考虑基于接口实现。具体字段含义见 "规则数据结构设计",其中 rule_id 为必填字段,rule_desc、create_time、modify_time 根据实际情况填写,params、code 则根据规则类型决定是否填写,如规则类型为1 ,则为基于参数的简单规则,则 params 必填,如规则类型为2 ,则为基于代码的复杂型规则,code 必填。以下给出俩种规则配置的例子:
- 简单规则配置

- 复杂规则配置

规则类型
针对简单规则和复杂规则,有不同的实现。
- 简单规则:逻辑变化不大,只有一些阈值或者系数发生变化的特征。针对简单规则,只需该规则的阈值或者系数封装到 Json 中,定时获取并更新 UDF 变量即可。
- 复杂规则:不限于系数和阈值变化,有增删改逻辑的变更。针对复杂规则,需要将代码逻辑封装在规则内,再使用代码生成框架例如 Janino 动态执行。由于复杂规则需要将代码封装成规则,每次规则变更需要提前进行调试,并且涉及到代码生成框架,会增加性能成本。所以针对固定场景的规则,建议将涉及到变更的参数封装成简单参数规则定时获取。针对不确定场景的规则再使用代码生成框架进行规则的生成。
规则数据结构设计
- rule_id :规则的唯一 id ,规则定义者根据 rule_id 更新规则,应用根据 rule_id 进行规则拉取。
- rule_desc :规则的描述信息。
- rule_type :规则类型,1 为基于参数的简单规则,2 为基于代码的复杂规则。
- params :简单规则的参数值。
- code :复杂规则的代码。
- ext :延伸属性,方便后续的功能扩展。
- create_time :规则创建时间,预留字段,暂无实际应用。
- modify_time :规则最新一次更新时间,在规则加载时,用于确认规则是否过期。udf 中保存最近一次规则的更新时间,若该时间小于数据库中的最新一次更新时间,则规则过期,加载规则,并且更新 udf 中最近一次更新时间字段。
{
"rule_id":"00001",
"rule_desc":"",
"rule_type":"1",
"conditions":{},
"params":{},
"code":"",
"ext":{},
"create_time":"",
"modify_time":""
}
规则加载方式
规则加载方式可选择基于 Flink SQL UDF 或者Flink DataStream RichMapFunction/RichFlatMapFunction 的方式:
- 基于 Flink SQL UDF 的方案,将规则存储在数据库中,在初始化 open 方法中创建数据库连接并首次拉取规则,在 eval 方法中定时判断规则是否需更新,如需更新则加载规则,并使用最新规则处理数据。
- 基于Flink DataStream RichMapFunction 的方案,将规则存储在数据库中,在初始化 open 方法中创建数据库连接并首次拉取规则,在 map/flatMap 方法中定时判断规则是否需更新,如需更新则加载规则,并使用最新规则处理数据。使用何种规则加载方式取决于原有的实时程序使用哪种技术实现,如使用 Flink SQL,则使用基于 Flink SQL UDF 实现规则加载;如使用 Flink DataStream,则使用基于 Flink DataStream RichMapFunction/RichFlatMapFunction 实现规则加载。
多场景基于动态规则的数据处理流程
实时报表新增维度动态规则实现
- 数据清洗、关联维度表 ,写 dwd topic。
- 抽象出一个统一的 Json 字符串类型字段,包含所有的新增维度,加维度时不需要再改变表结构。
- 将Json 字符串类型字段的生成逻辑封装成一个 Rule, 记录在数据库中,程序通过Rule Id 定时获取当前最新的 Rule ,并使用其处理数据,生成通用维度字段作为聚合维度落库。
- 针对加字段需求,只需要更新数据库里的代码逻辑便可实现。
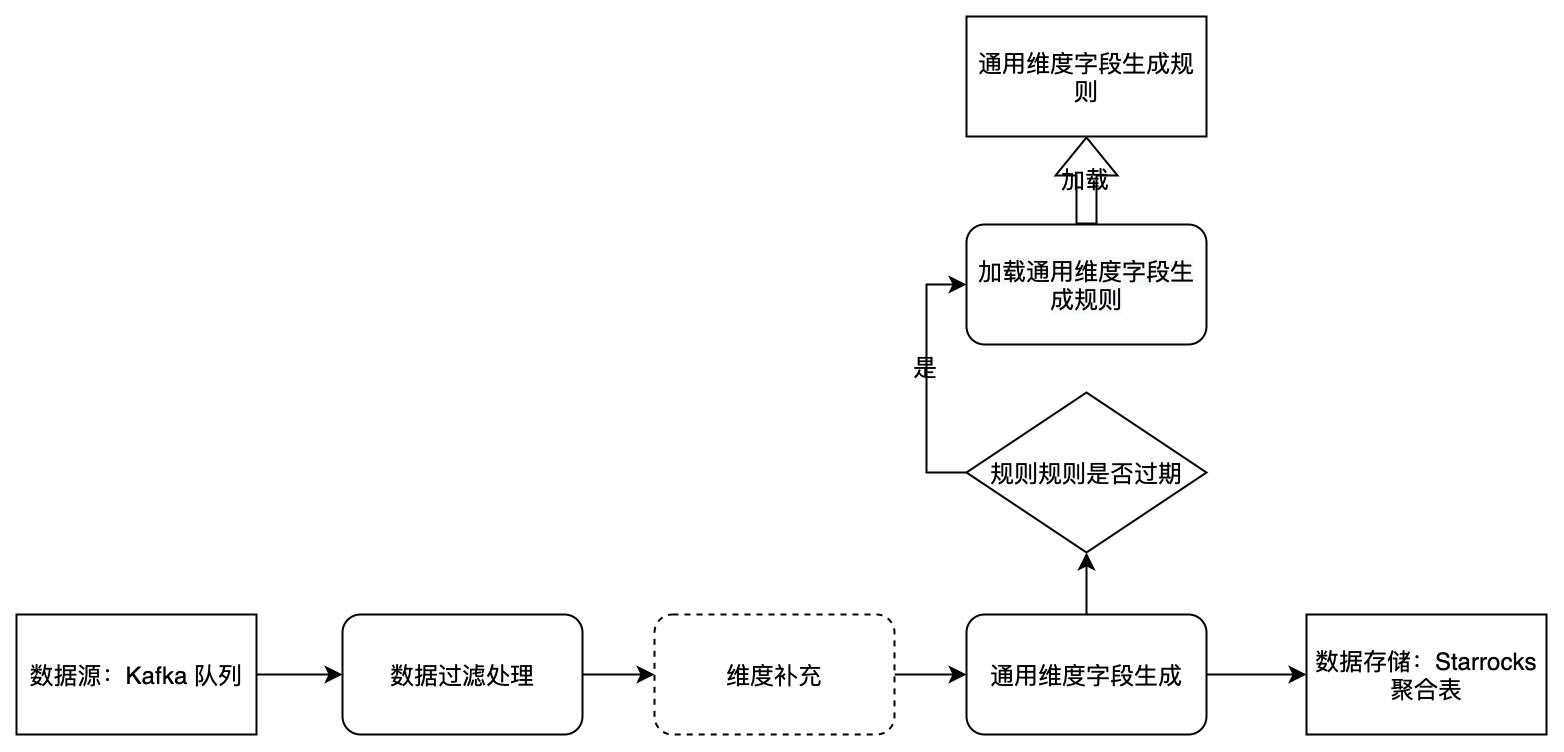
实时 ETL 逻辑变更动态规则实现
- 数据接入:使用 Flink SQL Kafka Source 时,将 value.format 设置为 raw。
- 数据处理:使用 Flink SQL UDF 加载规则,处理传入的参数 message。
- 数据分流过滤:使用 Flink SQL UDF 加载规则,处理传入的参数 message,出参为 bool 类型,根据出参判断本条数据是否传给下游。
- 数据存储:将处理后的 message 写入存储系统。
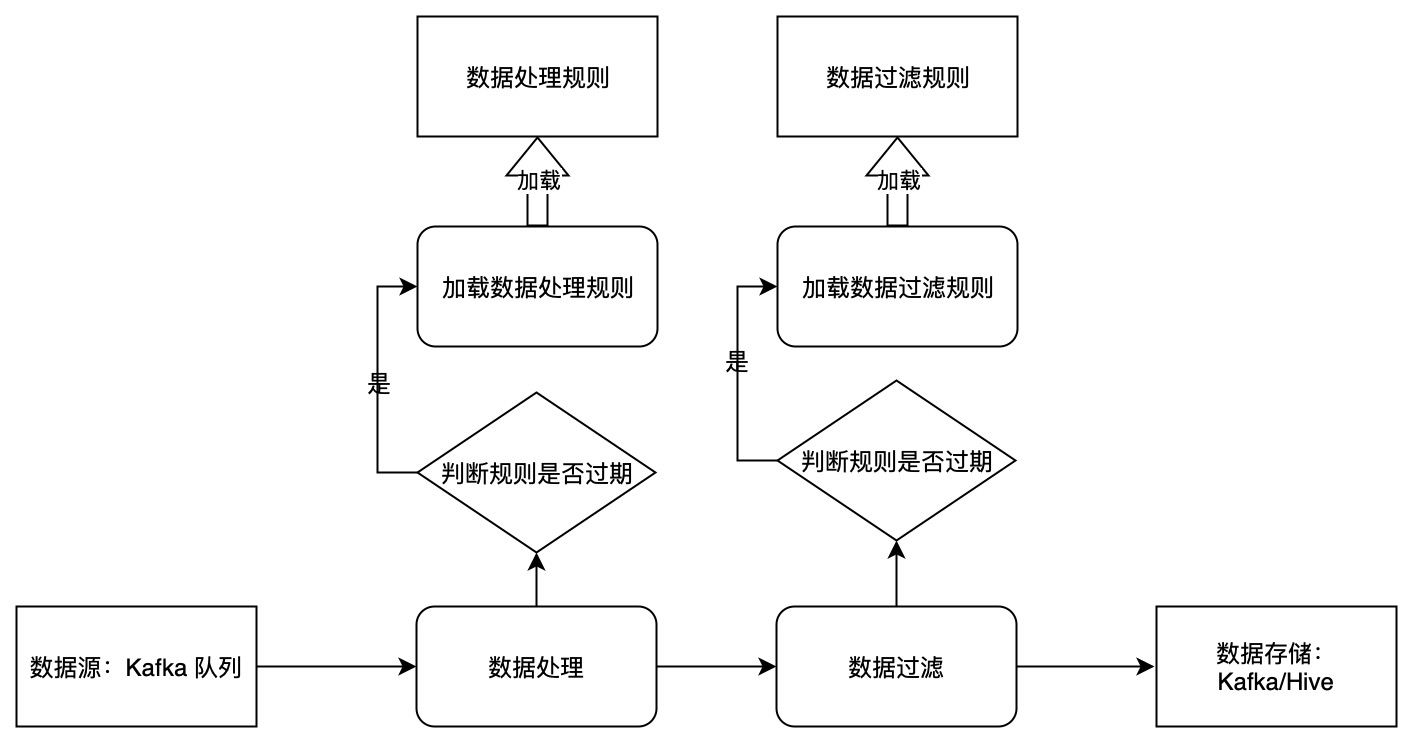
案例
需求背景
算法做 DSP 策略分析时,新增的场景需要使用新维度加以区分,所以加维度需求较为频繁。而且实时离线数据处理都需要新增维度,较为复杂。实时加维度需要经过:开发--->测试--->停止任务--->StarRocks 表新增字段--->代码上线--->启动任务;离线加字段需要经过:开发--->测试--->停止调度--->数仓/StarRocks 表新增字段--->代码上线--->启动调度。老的技术方案加字段不仅过程较多,容易出错,影响数据的正确性和及时性,而且每加一次字段都需要需求方、产品、开发、测试参与,周期较长。因此考虑引入规则配置的方式改善该流程。
工程实现
实时代码实现
实时规则引擎动态配置的过程为:实现 Flink SQL UDF 拉取配置,在 Flink SQL 中调用 UDF 生成通用字段 algo_dim,按 algo_dim 聚合计算出指标后写入 StarRocks。
Flink SQL UDF 实现
public class CodeRuleEngine extends ScalarFunction {
ScriptEvaluator se;
PreparedStatement preparedStatement;
Long modifyTime;
public void open(FunctionContext context) throws CompileException, SQLException, IOException {
se = new ScriptEvaluator();
String sql = "select code,modify_time from dim_rule_engine_acc_d where rule_id = ?";
Properties properties = new Properties();
properties.load(new InputStreamReader(this.getClass().getResourceAsStream("/jdbc.properties")));
String url = properties.getProperty("jdbc.url");
String username = properties.getProperty("jdbc.username");
String password = properties.getProperty("jdbc.password");
Connection connection = DriverManager.getConnection(url,username,password);
preparedStatement = connection.prepareStatement(sql);
se.setParameters(new String[] { "ad", "ext" }, new Class[] { String.class, String.class });
se.setReturnType(String.class);
preparedStatement.setLong(1,1);
ResultSet rs = preparedStatement.executeQuery();
String code = "";
while (rs.next()){
code = rs.getString(1);
modifyTime = rs.getLong(2);
}
se.cook(code);
}
public String eval(String ad,String ext) throws InvocationTargetException, CompileException, SQLException {
// 每隔一分钟加载规则
if(System.currentTimeMillis()/1000 % 60 == 0){
preparedStatement.setLong(1,1);
ResultSet rs = preparedStatement.executeQuery();
String code = "";
Long tmpModifyTime = 0L;
while (rs.next()){
code = rs.getString(1);
tmpModifyTime = rs.getLong(2);
}
if(tmpModifyTime > modifyTime){
se.cook(code);
modifyTime = tmpModifyTime;
}
}
return se.evaluate(new Object[]{ad,ext}).toString();
}
}
Flink SQL UDF 调用
SET table.exec.resource.default-parallelism = 20;
-- 设置ck目录
-- 设置checkpoint/savepoint恢复目录
-- 设置yarn上任务名称
-- 操作符链允许非shuffle操作位于同一线程中,完全避免序列化和反序列化(chaining合并,默认true,false便于观察)
SET execution.checkpointing.interval=1min;
--SET taskmanager.memory.process.size = '10g';
--SET jobmanager.memory.process.size = '10g';
CREATE FUNCTION JsonValue AS 'com.qm.udx.udf.JsonValue';
CREATE FUNCTION CodeRuleEngine AS 'com.qm.udx.udf.CodeRuleEngine';
CREATE TABLE kafka_source_ods_dsp_sdk_log (
request_id string,
ts bigint,
event_type bigint,
ext string,
advertiser string,
ad string,
app string,
pos string,
device string,
subr bigint,
nbr bigint,
proctime as proctime()
) WITH (
'connector' = 'kafka',
'topic' = 'xxxxxx',
'properties.bootstrap.servers' = 'xxxxxx',
'properties.group.id' = 'xxxxxx',
'scan.startup.mode' = 'group-offsets',
'scan.topic-partition-discovery.interval' = '180',
'format' = 'json',
'json.fail-on-missing-field' = 'false',
'json.ignore-parse-errors' = 'true'
);
CREATE TABLE final_result(
dt VARCHAR,
data_hour VARCHAR ,
data_min VARCHAR ,
......
algo_dim VARCHAR,
window_ts VARCHAR,
......
ad_exp_cnt BigInt
......
) WITH (
'connector' = 'starrocks',
'jdbc-url'='xxxxxx',
'load-url'='xxxxxx',
'database-name' = 'xxxxxx',
'table-name' = 'xxxxxx',
'username' = 'xxxxxx',
'password' = 'xxxxxx' ,
'sink.buffer-flush.max-rows' = '100000',
'sink.buffer-flush.max-bytes' = '300000000',
'sink.buffer-flush.interval-ms' = '5000',
'sink.properties.format' = 'json',
'sink.properties.strip_outer_array' = 'true',
'sink.max-retries' = '3',
'sink.parallelism' = '5'
);
CREATE TABLE dim_ad_group (
id DECIMAL(20,0),
conversion_id DECIMAL(20,0),
PRIMARY KEY (id) NOT ENFORCED
) WITH (
'connector' = 'jdbc',
'url' = 'xxxxxx',
'table-name' = 'xxxxxx',
'username' = 'xxxxxx',
'password' = 'xxxxxx',
'lookup.cache.max-rows' = '10000',
'lookup.cache.ttl' = '1h'
);
CREATE TABLE dim_asset_conversion (
id DECIMAL(20,0),
act_type INT ,
PRIMARY KEY (id) NOT ENFORCED
) WITH (
'connector' = 'jdbc',
'url' = 'xxxxxx',
'table-name' = 'xxxxxx',
'username' = 'xxxxxx',
'password' = 'xxxxxx',
'lookup.cache.max-rows' = '10000',
'lookup.cache.ttl' = '1h'
);
INSERT INTO final_result SELECT
cast(FROM_UNIXTIME(ts,'yyyy-MM-dd') AS String) AS dt,
cast(FROM_UNIXTIME(ts,'HH') AS String) AS data_hour,
cast(FROM_UNIXTIME(ts,'mm') AS String) AS data_min,
......
t3.act_type as act_type,
algo_dim,
cast(sum(case when event_type = '曝光' then 1 else 0 end ) AS BIGINT) ad_exp_cnt
FROM
(
select
request_id,
ts/1000 - ts/1000 % 300 as ts,
when event_type = 2001 then '曝光'
......
CodeRuleEngine(ad,ext) as algo_dim,
proctime
from
kafka_source_ods_dsp_sdk_log where nbr is null or nbr = 0 or coalesce(cast(nbr AS VARCHAR),'') = ''
) t1
LEFT JOIN
dim_ad_group FOR SYSTEM_TIME AS OF proctime t2
ON cast(ad_group_id AS DECIMAL(20,0)) = t2.id
LEFT JOIN
dim_asset_conversion FOR SYSTEM_TIME AS OF proctime t3
ON cast(t2.conversion_id AS DECIMAL(20,0)) = t3.id
where cast(FROM_UNIXTIME(ts,'yyyy-MM-dd') AS String) is not null and cast(FROM_UNIXTIME(ts,'yyyy-MM-dd') AS String) >= '2023-04-27'
GROUP BY
cast(FROM_UNIXTIME(ts,'yyyy-MM-dd') AS String) ,
cast(FROM_UNIXTIME(ts,'HH') AS String) ,
cast(FROM_UNIXTIME(ts,'mm') AS String) ,
TUMBLE(proctime, INTERVAL '1' MINUTE),
algo_dim
;
离线代码实现
离线规则引擎动态配置的过程为:实现 Sparl SQL UDF 拉取配置,在 dwd 层调用 UDF 生成通用字段 algo_dim,下层 (dwt/dm) 按 algo_dim 聚合计算出指标后写入 StarRocks。
Spark SQL UDF 实现
public class CodeRuleEngine implements UDF2<String,String,String> {
private static PreparedStatement preparedStatement;
private static ScriptEvaluator se ;
@Override
public String call(String ad, String ext) throws Exception {
if(preparedStatement == null){
synchronized (CodeRuleEngine.class){
if(preparedStatement == null){
String sql = "select code,modify_time from dim_rule_engine_acc_d where rule_id = ?";
Properties properties = new Properties();
try {
properties.load(new InputStreamReader(this.getClass().getResourceAsStream("/jdbc.properties")));
} catch (IOException e) {
throw new RuntimeException(e);
}
String url = properties.getProperty("jdbc.url");
String username = properties.getProperty("jdbc.username");
String password = properties.getProperty("jdbc.password");
Connection connection = null;
try {
connection = DriverManager.getConnection(url,username,password);
preparedStatement = connection.prepareStatement(sql);
} catch (SQLException e) {
throw new RuntimeException(e);
}
se = new ScriptEvaluator();
try {
preparedStatement.setLong(1,1);
} catch (SQLException e) {
throw new RuntimeException(e);
}
ResultSet rs = null;
try {
rs = preparedStatement.executeQuery();
} catch (SQLException e) {
throw new RuntimeException(e);
}
String code = "";
while (true){
try {
if (!rs.next()) break;
} catch (SQLException e) {
throw new RuntimeException(e);
}
try {
code = rs.getString(1);
} catch (SQLException e) {
throw new RuntimeException(e);
}
}
try {
se.setParameters(new String[] { "ad", "ext" }, new Class[] { String.class, String.class });
se.setReturnType(String.class);
se.cook(code);
} catch (CompileException e) {
throw new RuntimeException(e);
}
}
}
}
return se.evaluate(new Object[]{ad,ext}).toString();
}
}
Spark SQL UDF 调用
INSERT OVERWRITE TABLE dwd.dwd_dsp_aggs_log partition(dt='${input_date}',data_hour='${data_hour}')
SELECT
......
CodeRuleEngine(ad_info,ext) as algo_dim,
......
FROM
ods.ods_cc_dsp_log t1
LEFT JOIN
(select * from ods.ods_dsp_pixiu_dsp_ad_group_acc_h where dt = '${input_date}' and data_hour = '${data_hour}') t2
ON get_json_object(t1.ad_info,'$.group_id') = t2.id
LEFT JOIN
(select * from ods.ods_dsp_pixiu_dsp_asset_conversion_acc_h where dt = '${input_date}' and data_hour = '${data_hour}') t3
ON t2.conversion_id = t3.id
where
t1.dt = '${input_date}' and t1.data_hour = '${data_hour}'
问题和改进
当前主要存在以下几点问题:
- 当前 UDF 输入参数只支持来自日志,不能来自维表。
- 只支持新增维度,不支持新增指标。
- 程序间规则加载代码不能共用。
针对以上几点问题,计划从如下方面进行改进:
- 使用 dwd 层 Kafka topic 大宽表表,上游任务关联维表写 dwd 层 topic,下层任务读 dwd 层 topic 进行指标计算, 当新增的维度需要关联新维表时,只需重启上层任务新增维表,下游任务更新规则即可。
- 当前新增维度采用了大 Json 字段存储所有新增维度,指标需要新建字段独立存储,所以可以采取预留字段的方式实现。
- 抽象加载过程中的通用代码,支持规则 id 以参数的形式传入 UDF 或者 RichMapFunction/RichFlatMapFunction 。